The Economist’s Technology Quarterly this last week was entitled “Artificial Intelligence and It’s Limits.” It’s worth a read or two. While it is meant to address the limits of AI in general, much of it is focused on the limits of Machine Learning, a particular branch of AI. In short, the point is that while firms such as PWC, McKinsey, and IBM have predicted that AI will add something between $8-13 trillion to the global economy by 2030, there are issues that companies are encountering as they try to enter this “AI Promised Land.”
The Economist’s article laid out why algorithm-, data- and processing-rich firms such as Google led the way and saw such profound changes, but also why others have not found such dramatic transformations. In short, AI relies on ever-advancing algorithms, ever-improving computer processing on which to run the algorithms, and ever-increasing data from which the systems can learn. As a result, it is the companies that find themselves at these crossroads or those that can reimagine their business processes to rely on these headwinds that look to benefit the most from AI, and in particular Machine Learning. So with this said, I’d like to delve into a second interview in our review of DARQness (Distributed Ledger, Artificial Intelligence, Virtual and Augmented Reality, and Quantum Computing) with an interview with an old friend of mine, Kelly White, who is someone who did use AI tools to reimagine and address the pressing problems of his domain – cybersecurity.
Cybersecurity, AI and Machine Learning
About six years ago, Kelly White and I, who went to high school together in Oregon, found ourselves together again here in Utah. It didn’t take long for us to pick up old habits, like watching movies and working out together. So a few months after we started spending time together, we found ourselves at a new Luc Besson joint called Lucy, which is along the line of Besson’s other movies La Femme Nikita and the Fifth Element, about the evolution of mankind via womankind (something that I like, especially considering that I’m the father of three daughters). For those of you who haven’t seen it, in the movie Scarlett Johansson, the eponymous titular character Lucy, overdoses on a nootropic drug like those used by so many elite programmers in Silicon Valley. She then evolves rapidly and eventually merges with computers becoming something akin to a god (props to Ray Kurzweil and the Singularity). It was at the end of the movie that Kelly and I sat in the theatre and talked about our own versions of the merging of man and machine.
Our discussion was particularly relevant because Kelly had gone to work in IT security and how he had a vision of coding up software that would serve what he saw as a dire need. As we hung out (and worked out) that summer, we talked about the vision that was forming in his own mind. I did what I could to help but to be blunt, there is only so much anyone can do for another person, with most being limited to moral support. Then one day it happened. No, not “the Lucy thing,” but rather that Kelly got a prototype of his software running and then got General Catalyst and a few other groups to back him in bringing it to market. It was beautiful and I’m just happy that I could see it unfold. That idea that became a company, RiskRecon, was recently sold to MasterCard.
As Kelly and I spoke recently, we found ourselves talking about the parallels in IT Security and Finance, so I asked Kelly to be my next interviewee and asked him to talk about AI. What follows is an excerpt from a couple of hours of conversation and a summary of what can only be described as Kelly’s life’s work. It is my next entry on DARQness because Kelly used, and will continue to use, Machine Learning in his business (the “A” in DARQ, for “Artificial Intelligence”).
Kelly White, RiskRecon and Tools of the Trade
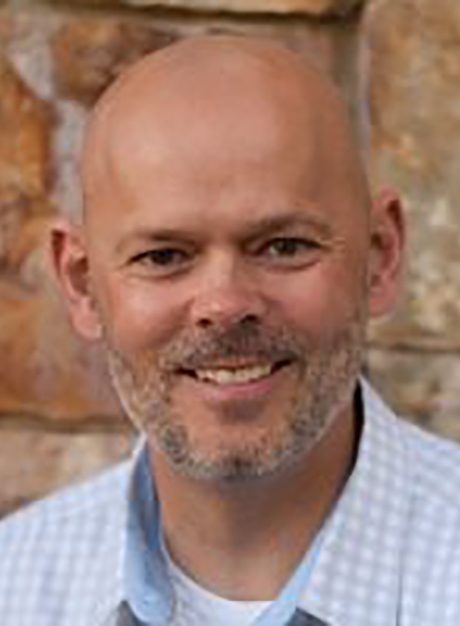
James: Kelly, tell me about Cybersecurity and what you do in the area?
Kelly: I suppose the most common way that cybersecurity is understood is miscreants attempting to compromise systems and professionals working to prevent them from breaking in. That is certainly part of it. However, cybersecurity is better represented as a discipline of risk management wherein risk is the probable frequency and magnitude of loss. Cybersecurity risk management is the discipline of allocating limited resources to achieve desired risk outcomes. As such, it isn’t unlike other risk disciplines in industries such as finance and insurance.
What do I do in the area? I run a company, RiskRecon, that provides technology that helps cybersecurity risk professionals better understand and act on their cybersecurity risk.
James: And how do you help cybersecurity risk professionals better understand the risks facing them and then act on those risks?
Kelly: Good risk decisions are founded on good data and analytics. And because the cybersecurity risk landscape changes so quickly, the cycle of data collection and analytics has to occur very rapidly. Done manually, the collection of data and the necessary analytics to understand the risk landscape and act on that risk is too slow and resource-intensive. My company, RiskRecon, has automated much of the cybersecurity risk data collection and analytics, enabling cybersecurity professionals to focus on the higher-order activities of risk decision and action.
I and several members of my executive team have done cybersecurity risk management for decades for a wide range of industries, so it wasn’t like we were starting from nothing. Risk management is about understanding probable frequencies and magnitudes of loss and allocating resources to maintain your risk exposure at a tolerable level. Our software solves much of that problem automatically – assessing a company’s risk state. We can automatically pinpoint areas of strength and weakness and where there is the greatest value at risk and the highest probable loss likelihood.
James: Could you talk about Artificial Intelligence in this context?
Kelly: I personally chafe a bit at using the term “Artificial Intelligence.” In reality, what we do is use a variety of Machine Learning models, which are just software tools that people often use under the heading of “Artificial Intelligence.” We use a range of Machine Learning models to automatically discover the systems that companies operate on the internet and to determine the value at risk of each system – the types of data the systems collect and the transactions they support. Tracking the systems and their data/function is a prime use of machine learning models because the systems, their functions, and their security state change so rapidly that it is impossible for humans to keep up.
James: Could you talk about this term “Value at Risk”, this is a big topic for us in finance.
Kelly: In cybersecurity, we use the term “Value at Risk” to mean the types and volumes of data and transactions that a system supports. For example, an obscure brochure site has very low value at risk relative to an online trading portal that collects and stores massive amounts of sensitive data and that provides functionality for moving money around the financial system. It is useful to break “value at risk” down into two sub-parts – inherent risk and residual risk. Inherent value at risk is the expected frequency and magnitude of loss without factoring in controls; think about sticking the digital pile of gold on a publicly accessible S3 bucket for anyone to take. Residual value at risk is the expected frequency and magnitude of loss factoring in controls. Now think about sticking that pile of gold in a digital Fort Knox. Understanding value at risk, both inherent and residual risk, is the foundation of good risk decisions. Viewed another way, that is what RiskRecon does – helps organizations understand their inherent and residual value at risk at an enterprise and at a system level. Our data directs professionals to areas where the residual risk is too high and additional control is needed.
James: Is RiskRecon and innovations like it in cybersecurity going to decrease employment in the area?
Kelly: No. There is a massive shortage of cybersecurity professionals, which has created what I call a cybersecurity “wealth gap”. The big rich companies have robust, talented cybersecurity teams. Other companies? They don’t. They can’t afford to build the teams or they simply can’t attract the talent. There simply aren’t enough professionals to go around. This has created a real problem. A small number of companies are really secure, while the majority are not. Technologies like RiskRecon help solve that “wealth gap” by enabling companies to understand and act on their cybersecurity risk without having to have a large staff of cybersecurity professionals. Certainly, technology alone is no match for a talented team armed with good tech, but it does help close some of the wealth gap.
James: Could you close with a bit more about Machine Learning and how you use it RiskRecon?
Kelly: I often listen to this MIT Podcast on Artificial Intelligence and a common theme is that complexity has just really gotten away from us. That is the case with cybersecurity. Humans cannot alone keep up with the massive proliferation and complexity of the Internet, let alone their own company. Machine Learning algorithms can keep up. Fortunately, cyber is a very data-rich environment which is what Machine Learning models need – lots and lots of rapidly changing data. We’ve trained models to solve a subset of the problems that risk professionals have long solved manually. There is a lot of greenfield opportunities for Machine Learning algorithms to help solve cybersecurity challenges. I am super excited for what comes next, both from RiskRecon and other algorithm-centric cybersecurity companies.
Update on the ULISSES Project
Kelly’s interview highlighted two of the three things that are most important with AI: better algorithms and better data. In the next entry, I will discuss the third leg of the AI stool, which is achieving better processing in the face of the effective cessation of Moore’s Law and how we are addressing those issues in the ULISSES Project. This said, I want to make it clear if I already didn’t, I’m a firm believer in the future of AI and what can be done in finance, provided all three legs of the stool are improving together (algorithms, computer processing, and data). Along these lines, I’d like to announce a new member joining us in our efforts at the ULISSES Project, Eric Jensen of Causality Link.
Causality Link is an AI-based (Machine Learning focused) company out of here in Salt Lake, led by Pierre Haren and Eric Jensen. Pierre is the former CEO of ILOG (sold to IBM) and after their acquisition, he stayed on to run the business side of the Advanced Analytics and Cognitive Sciences for IBM (the group that included Watson). As far as Eric, he has a long history here at the University of Utah, in particular helping the Games Program develop their first curriculum and then some interesting work in EdTech. While the AIAX Initiative previously focused largely on the axiomatization framework, we are now expanding into a new and exciting project bringing together that more “symbolic” perspective with Machine Learning technologies, all hosted on the FlexAxion platform. We look forward to working with Eric and pushing forward in this new direction for the AIAX Initiative. Expect interesting updates with it going forward.